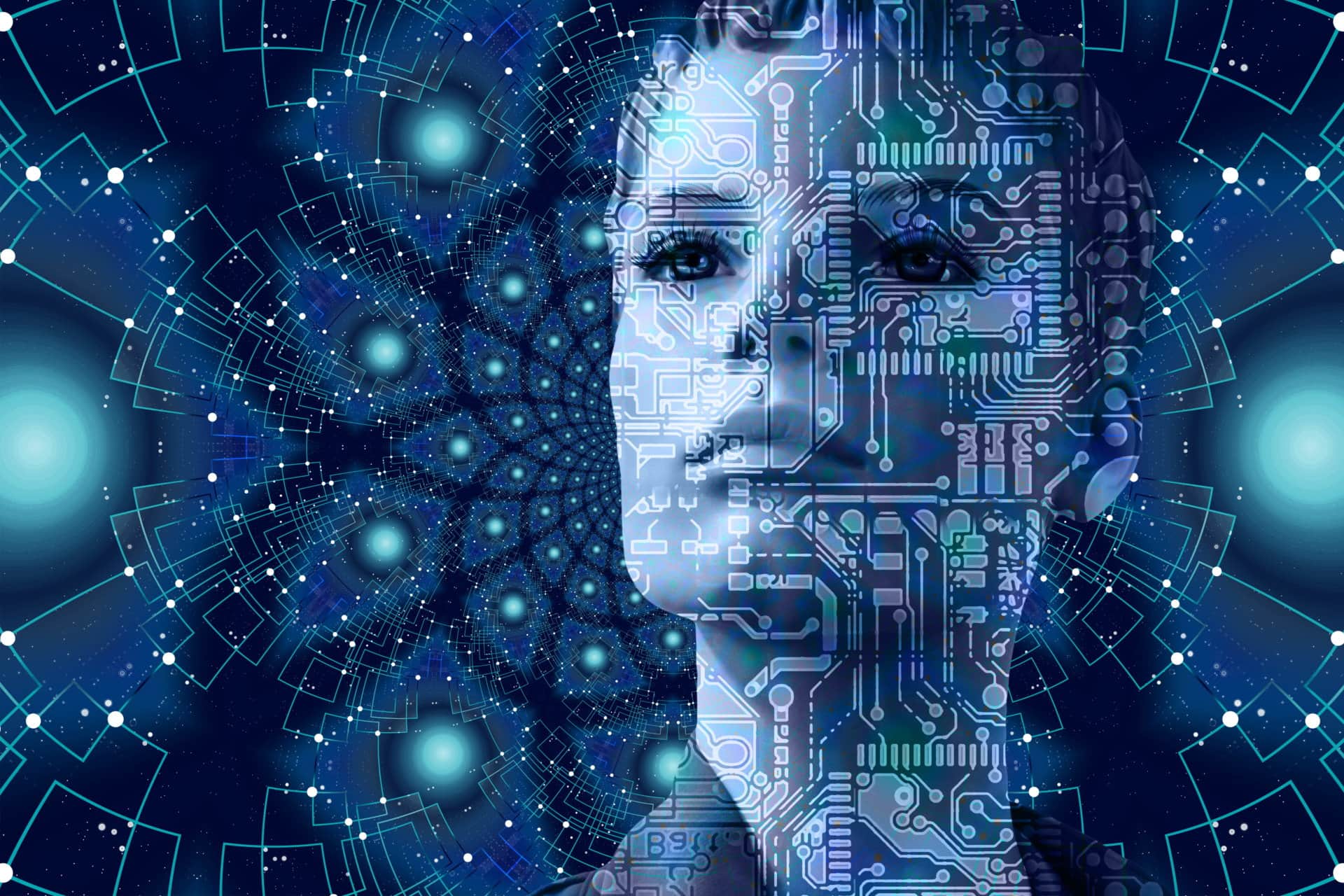
Upskilling, reskilling, and rightskilling (getting the right skills to the right place at the right time) is a top priority for the C-suite, human resources (HR), and learning and development (L&D). The skills conversation has accelerated since the onset of the COVID-19 pandemic, as organizations scrambled to rapidly reskill and upskill their workforces. As the interest in skills has intensified, workplace technology vendors have taken notice, and have developed artificial intelligence (AI) capabilities to address some of the more difficult, persistent challenges. With AI, there are new possibilities to power a skills ecosystem that is strategic, dynamic, adaptable, and personalized.
Only 33% of skill development programs achieve their desired objectives and business impact.
– McKinsey, The Skillful Corporation
In McKinsey’s Skillful Corporation Survey, 78% of leaders said skill-building is important to their long-term growth. In the same survey, 87% have current skill gaps or expect to in the next few years. Yet they report only 33% of skill-building programs succeed at achieving the planned objectives and business impact, meaning 2 in 3 of these programs fail.
Why is something so important, so critical to the C-suite so difficult to execute?
Why do so many skills programs underwhelm expectations and fail to generate positive results?
Successful skills strategy requires overcoming a series persistent, complex challenges. As artificial or augmented intelligence (AI) capabilities have expanded, they’ve changed the nature of what’s possible. And, what is possible is an AI-powered skills ecosystem is more dynamic and scalable than ever before. The diagram below shows some of the core capabilities, including:
- Aggregating and analyzing multiple sources of internal and external skills data.
- Maintaining employee skill profiles in real-time.
- Defining a skills architecture (ontology) that defines how skills are named, how they relate to each other, and how they map to roles and jobs.
- Generating real-time skill supply and demand insights.
- Providing suggestions and recommendations on how to close skill gaps, including learning opportunities, coaching, internal mobility, and stretch assignments.
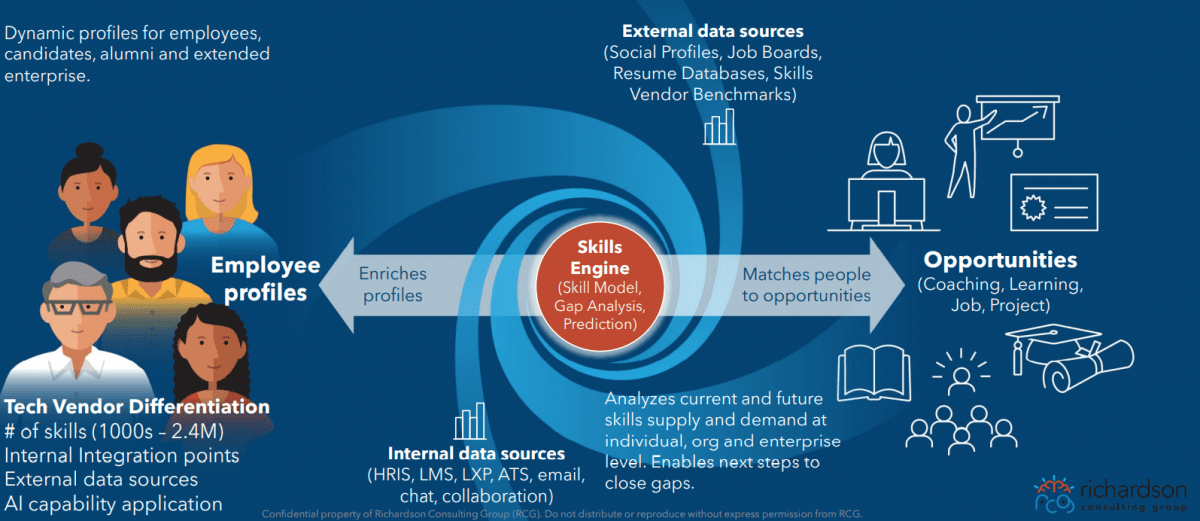
To understand these challenges in more depth, and the ways in which AI is changing what is possible, consider:
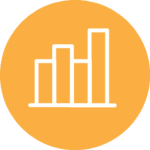
Skill Data
The most common skills data in most organizations is self-assessment data. Once or twice a year, employees self-rate on a scale on skills they select, or on skills they are asked to rate based on their role(s) in the organization. In many organizations managers will validate the employee’s skills and ratings, and/or complete what is called a 180-degree evaluation by rating the employee on the same skills and scale. Some organizations will use 360-degree or 720-degree evaluations, which include peer and/or customer ratings.
An issue with this approach is that the data is based on human judgement, and thus introduces the potential for several forms of bias. Another is that the data can become stale or less relevant over time. Updating these ratings takes time, regular reminders to update ratings, and leadership support.
Some other common sources for skills data are:
- Tracking learning activity mapped to employee skill development.
- Skill assessments to get a more performance-based view of skills.
- Certification programs to validate skills.
These have the advantage of producing high quality data, but are also high effort and high cost, which limits their scalability.
A further challenge with skills data is that it is often stored in multiple systems. Employee skills data may be in multiple internal systems including:
- HR information system (HRIS)
- Human capital management (HCM) system
- Applicant tracking system (ATS) used by recruiters
- Project management systems
- Workflow management applicaitons
- Learning management system (LMS)
- Learning experience platform (LXP)
- Custom and/or third-party skills libraries
- Internal social collaboration platforms like Slack and Teams
- Contingent workforce management systems
Still more employee skills data may exist outside the organization on social media sites like LinkedIn, external learning platforms like Coursera, or in online communities like Stack Overflow.
AI addresses this problem by continually ingesting and analyzing massive volumes of skills data from multiple internal and external sources, then using that data to create a dynamic skill profile for each employee. This profile is continuously updated based on new data from these sources.
AI then enriches those profiles with other data it has for that individual. It can infer skills employees have based on groups they belong to, where they went to school, other places they’ve worked or other roles they’ve had. Or if it uses unstructured sources like email, Slack or Teams it can see, for example, that someone is answering a lot of questions about a topic and infer that they have one or more skills related to that topic.
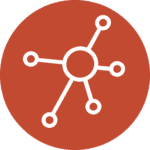
Skills Architecture
Starting in the 1980’s, companies started building competency models, that included knowledge, skills and abilities (KSAs). They then mapped those competencies to jobs, and then used those competencies to write job descriptions, rate job performance, create learning programs and build career paths. This helped us make these processes much more performance-based, particularly as knowledge work grew in importance and in the share of the workforce.
Knowledge workers (ex. programmers, engineers, architects, scientists, researchers, designers, etc.) often do not organically have as many observable, measurable performance indicators as part of their roles as those engaged in manufacturing work, sales, or front-line service work. These roles often are not as strictly output-based, or as easily tied to top and bottom-line business metrics like revenue, operating expense and profitability.
As workforce trends like hyper-specialization, automation, project-based work, new ways of working, and technology innovation accelerated, these models became more difficult to manage and maintain. For some technical skills, their “shelf-life” or “half-life” has, according to some research, shrunk to an average of 5 years.
The half-life of jobs and roles is also shrinking. Across industries and roles, the rate of change of both the work that needs doing and the way work gets done makes maintaining a static competency model or skills library difficult. Maintaining the relationship of that model to individual jobs and roles is another layer of complexity and point of failure.
The traditional static, manual models are cumbersome to build and maintain, and they tend to collapse under their own weight over time. Even if you are buying someone else’s model, it starts to become outdated the moment it is published.
AI addresses this by continually ingesting and analyzing massive volumes of skills data from internal and external sources, then using that data to create a dynamic skills architecture. These data sources include:
- Structured internal sources like your people and learning systems, as well as internally-developed, and/or third party skills libraries.
- Unstructured sources like email, chat, and internal collaboration platforms.
- External sources like LinkedIn, job sites, resume databases, government sources, etc.
AI processes this data in real-time to create a dynamic skills architecture with thousands or even millions of skills, how they relate to each other, and how they relate to roles and jobs. These systems constantly crawl both your internal sources and external sources for more data, updating the model as it learns more.
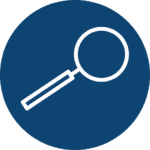
Skills Insights
What skills do we have in our organization?
What skills do we need right now?
What skills are trending in our industry, or in our function?
What skills will we need in 3 months? In 3 years?
These are important, strategic questions every organization wants to answer, but if skills data and skills architecture are a challenge, gaining even the most basic level of insight will be difficult and expensive. Traditional approaches require manual aggregation and analysis of internal and market data. The process is labor-intensive and difficult to scale, and provides only a point-in-time, snapshot view. If another snapshot is needed in 6 months or a year, the data aggregation and analysis must be repeated. Forecasting future supply and demand is guesswork.
As with data and architecture, AI can take a much more robust and dynamic approach based on processing high volumes of internal and external skills data. Current supply and demand and forecasting can be accomplished based on hiring trends, trends for posted vs. applied for postings, what people are learning and a host of other factors both within your organization and in the overall market. AI can also analyze skill surpluses and deficits across departments within the organization, as well as within your employee, hiring and contingent labor skills reservoirs.
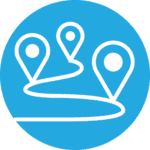
Skills Action
Knowing what the skill gaps are at an individual, organization or enterprise level is a critical step, but the next is to determine what to do about it. AI can make enterprise-level recommendations on buy/build/borrow strategies to close gaps based on cost, availability, urgency and expected duration of the gap. Strategies to close skill gaps include:
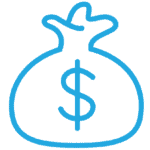
Buy
A buy strategy hires someone new into the organization. For hiring professionals, AI can rank and recommend candidates for open positions based on their current skills, as well as their potential to develop new skills, while factoring in diversity goals, and reducing decision bias.
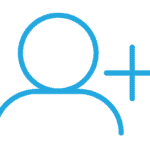
Build
A build strategy upskills or reskills someone already in your organization- For employees, AI can recommend learning, career, mentoring, coaching, internal mobility and other development opportunities based on individual skill goals and gaps. It can also recommend skills to develop based on trends within the organization and the overall job market to target skills that are or will be in demand.
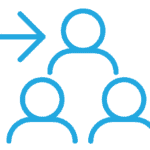
Borrow
A borrow strategy brings on contingent workers. It is possible to apply the same skill data aggregation and inference to a contingent workforce. Contingent workforce management systems may contain skills data that can be enriched with external data to build more complete profiles. Those profiles can then be matched to open project opportunities.
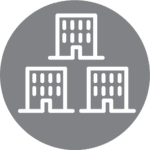
Vendor Landscape
There is no single technology solution that fully addresses every challenge. Several of our clients are using advanced skills functionality from our partners at Eightfold.ai and Degreed. All the major HR and learning tech vendors are building skills cloud capabilities including Workday, Oracle, SAP, Cornerstone, and EdCast. Others vendors in this space include IBM, Microsoft, SkyHive, Gloat, Hitch Works, StarMind, TechWolf, and many more.
These systems can be connected through application programming interfaces (APIs), and/or through other means of data and functional integration. The right-fit solution and set of integrations will differ for each organization based on a range of factors including:
- The business problems they are trying to solve with skills.
- Their current skills strategy and technology ecosystem.
- Available resources and capacity to acquire and adopt new technology.
AI can make skills data, architecture, insights, and action more robust, dynamic, and scalable, but it is just one piece of the skills puzzle. Successful skills strategy requires:
- Setting clear goals and priorities based on solving business problems.
- Creating a skills culture that values and promotes continuous skill development.
- Embedding a skills mindset across the organization.
- Adding a skills lens to business processes and ways of working.
If your organization is new to the skills journey, start with the business problems you are trying to solve, with building skills mindset and culture, and assess the how well the skills capabilities of your current technology supports your goals before considering new tools and platforms. If you’ve laid a strong strategic skills foundation, reach out to your core HR, learning, and work tech vendors to understand current skill capabilities that might be under-utilized, as well as to understand their roadmap for skills. And if you need some assistance or direction, contact us, and we’ll be happy to help.